Data collection has been democratized over the past twenty years. It’s easier and cheaper than ever to build a survey or a form, collect data, and analyze it. In just a few minutes, anyone with minimal surveying experience can build a short web survey, email the link to a contact list, and look at live data coming in on pre-built dashboards. Don’t have a list? No problem! You can pay $1 (or less) per response from Google Consumer Surveys, Amazon Mechanical Turk, or any number of online survey panels. With just a few hundred dollars, you can get answers to your heart desires in under 24 hours.
Democratization has its dark side as well. What was once a time-intensive task for people with advanced degrees is now often relegated to junior staff with minimal survey design training or experience -- trust me, I was one of these people at my first job out of college. Younger employees tend to be most eager to (and assigned to) using new technologies. Online software has made it simple to rapidly field low-quality forms, surveys, and other modes of data collection. It’s no surprise that the blogs of online survey vendors are littered with posts about the most basic survey design advice, such as asking one question at a time.1 Paradoxically, the low price tag means that organizations pay less attention to the design of these tools. If you miss something, you can always add another question or collect more data later.
Now there’s hardly a consumer or government interaction these days that doesn’t ask me for a review or a “short” survey. We’re all swimming in requests for data. We’re asked to provide information on tools that are too long, too intrusive, and too confusing. There’s even an industry term for the feeling of being over asked: “survey fatigue.”2 It’s an apt name. I’m personally tired of being inundated with requests -- and poorly designed ones are the most enervating.
It wasn’t always like this. The other day, I was looking back on how data collection was done before modern computing in the 1940s and 1950s. Most folks who haven’t graced a sociology classroom or survey design course probably haven’t heard of Paul Lazarsfeld and his colleagues at the Bureau of Applied Social Research at Columbia University. They were public intellectual giants in their day and pioneers in large-scale and rigorous survey research.
Back then, collecting data was expensive and very time-consuming. In-person interviews, focus groups, and mail surveys were the primary way to collect information.3 There was some data collection by phone, but more than one-third of the population didn’t have access to phones, and even those who did would often share lines.4 That said, being asked for an opinion was a bit of a novelty (and rarity) and response rates for a typical survey were north of 90%!5
Information was hand-recorded and stored on punch cards, which could be used by card sorting machines for “quick” tabulations. The IBM cards, the standard of the day, could hold about 80 columns worth of data. Those 80 columns aren’t not just for questions asked; that’s for any information you would need. Location, time, interviewer, and anything would have to be represented with some combination of holes in order to be used by the card sorters and readers. To illustrate how this worked in practice, one seminal multi-year study in 1948 by the Bureau of Applied Social Research of over 1,000 voters in Elmira, New York only asked about 40 questions in large part because of these constraints.
Analyzing the data, of course, was really hard too. You could create simple tables with counts of variables produced by punch card sorting machines or do some regression math by hand-programming early computers.6 And it’s not like these machines were at everyone’s desk where you could run analysis after analysis with the stroke of a key. They were expensive machines that were in high demand. You had to book time on these machines far in advance and your analysis would be run in batches with a bunch of other folks.7
You might think that these constraints basically made it nearly impossible to do high-quality work. But you’d be wrong. The research done at Columbia fundamentally changed how we understood social and political influence for decades. They developed ideas you likely have heard of, such as so-called “key opinion leaders,” social influence on people’s political views, and how new innovations spread. The questions they were looking into are still ones that social scientists are debating to this day.
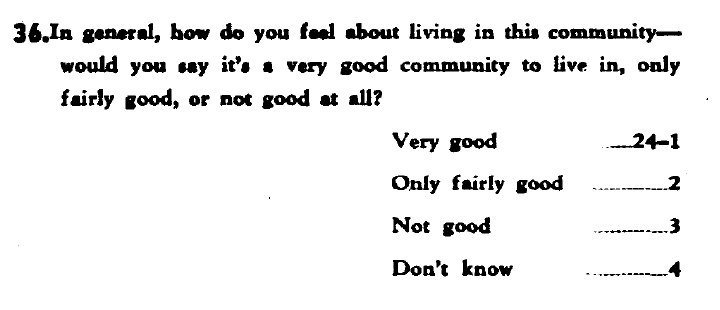
Not only are broader questions they asked still relevant but their questionnaires -- nearly 75 years later -- still hold up pretty well. The questions are simple to read and understand. They don’t seem redundant or irrelevant to the task at hand. And keep in mind, this is without the benefit of modern standards and norms for doing these types of studies -- they were the pioneers. Sure, if you were re-running the study today, you’d do a few things differently. But that’s true about pretty much any survey.
How were they able to do great work with these limitations? My view is that the constraints actually helped them improve their data collection process. They were forced to spend a lot of time on planning precisely because they were severely limited in the number of questions they could ask and what answers they could collect. There were no “throw in” questions -- each needed a justification. The technological limitations also forced them to think about the analysis ahead of time. They had to organize data on punch cards so the sorting machines could produce the tables they wanted.
It’s so easy now to add just one more question on an online form or survey. Gone are the tough deliberations over what’s in and what’s out. Just imagine Lazarsfeld and colleagues in uncomfortable sport coats with patches on their sleeves arguing around a table with a dusty chalkboard. Adding another question on a web form doesn’t mean buying more expensive punch cards or adding additional postage to your budget for a mail survey. It doesn’t mean making your data processing a ton more complex. The conversation is no longer “why do we need this question instead of that one?” and more “why not ask this in case we need this later on?”
This shift means that designers of data collection tools increasingly downplay (if not entirely discount) the imposition on the ones completing these forms. The time and energy it takes to read and digest survey questions or a form is pretty much unchanged since Lazarsfeld was surveying. From a user’s perspective, there’s not too much of a difference between clicking a button versus filling in a paper form. The burden on data providers wasn’t really a huge issue in the 1940s or 1950s when there was a low volume of requests. Taking a survey was kind of a novelty. But now that we’re flooded with requests for information, we’re becoming more sensitive to these things.
The proliferation of information requests is accelerating long-standing trends of declining response rates to data requests across modes.8 Phone surveys, still the “gold standard” for polls today, have gone from about 1 in 3 respondents answering in 1997 to now closer to 1 in 20.9 Even if you get someone to start a survey, completion rates are lower as well.10 There’s no sign of this trend abating, especially with growing concerns about data privacy.
This sounds like mostly doom and gloom, but I don’t think that’s the case at all. I believe there’s room for organizations to design data collection tools and processes that differentiate them from others. Tools that are respectful of the time and situation of users. Tools where data providers have some transparency on how the information will be used. Tools that energize instead of enervate. The past has a lot to teach us here. We shouldn’t go back to the days of punch cards, but I do think there’s a lot to learn from how people thought about data collection when it was more difficult to do. And there’s no reason we can’t integrate those learnings with advances in technology to ask better questions that collect more useful data.
The purpose of this project is to share some of my ideas -- and elevate other smarter folks out there from whom I’ve learned. I’ll include both the successes and mistakes I’ve made as a practitioner mostly working with governments and nonprofits to more efficiently and inclusively collect data as well as in academia. My goal for this series is to start a practitioner-oriented discussion on how we can make data collection better. I want to take a broad view of the entire data pipeline, from designing tools to presenting results. Many readers will disagree with many of my suggestions; that’s ok with me. My primary goal is to get people to reflect and consider challenges to data collection that may not always be top of mind.
We should always be asking ourselves and our teammates “how exactly does this request tie to our current goals?” If you can’t make that justification, you either don’t have clear goals or don’t have a good reason for including asking.
So-called “double-barrel” questions are pretty common for new survey programmers. For example, CultureAmp, a survey company, asks on their employee engagement survey the following: Generally, the right people are rewarded and recognized at [Your Company]. You could imagine that being rewarded (getting actual compensation or promotion) is different than getting recognized (getting public attention). So it’s confusing to survey takers.
https://www.qualtrics.com/blog/avoiding-survey-fatigue/
Groves, R.M., 2011. Three eras of survey research. Public opinion quarterly, 75(5), pp.861-871. https://academic.oup.com/poq/article-pdf/75/5/861/5184432/nfr057.pdf
https://www.technologyreview.com/2012/05/09/186160/are-smart-phones-spreading-faster-than-any-technology-in-human-history/
Groves, R.M., 2011. Three eras of survey research. Public opinion quarterly, 75(5), pp.861-871. https://academic.oup.com/poq/article-pdf/75/5/861/5184432/nfr057.pdf
For some old examples, see:
https://www.computerhistory.org/revolution/punched-cards/2/12/97
http://www.columbia.edu/cu/computinghistory/sorters.html
For an idea of this, here’s an amazing interview with an MIT professor on some of the bottleneck issues:
https://mitadmissions.org/blogs/entry/mit-computer-timesharing-in-the-1960s/
Nonresponse in Social Science Surveys: A Research Agenda https://www.nap.edu/catalog/18293/nonresponse-in-social-science-surveys-a-research-agenda
https://www.pewresearch.org/fact-tank/2019/02/27/response-rates-in-telephone-surveys-have-resumed-their-decline/
Nonresponse in Social Science Surveys: A Research Agenda https://www.nap.edu/catalog/18293/nonresponse-in-social-science-surveys-a-research-agenda
Loved the post :-). Poking the bear here deliberately -- what do you think of attempts like NPS to reduce survey load but still get at something "valuable"?
Cool essay, and the easily ignored "Please take this short survey" is spot-on. But it seems like a commons problem of sorts. If the ocean of survey respondents is being drastically overfished, how do you get the fishermen to let up? Especially if you're in a situation where sometimes quantity of response can make up for quality (I'm thinking the Netflix/Pandora/Reddit thumbs up or thumbs down). The incentives seem pretty skewed...